Self-learning Caches
Caches in modern storage systems are static and unable to learn and adapt. There is strong evidence that the application of self-learning into storage caches can unlock huge improvements in cost, efficiency, performance and predictability. Cache are inherently nonlinear systems making their tuning an exercise equivalent to solving a maze in the dark. This is precisely why the application of self-learning techniques can yield such a huge improvement against the static baseline.
In this talk, we will begin by providing an overview of the opportunity that self-learning caches promise by looking at some case studies. Irrespective of whether the cache is in a storage system, database or application tier, no two real workload mixes have the same cache behavior so we will look at several different real examples. Based on research recently published (USENIX ATC '17), we will review techniques for online, real-time self-learning caches and describe how they achieve huge gains.
Learning Objectives
- Why static caches leave so much performance on the floor
- What is a self-learning cache and how does it automatically adapt to new workloads
- The efficiency, QoS, performance SLAs/SLOs and cost tradeoffs that self-learning caches enable
- The broader application of self-learning cache technology to multi-tier and multi-tenant systems
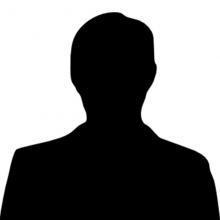